Amazon Bedrock は、基礎モデル (FM) を使用して生成 AI アプリケーションを構築および拡張する最も簡単な方法です。 FM は膨大な量のデータに基づいてトレーニングされており、それらのデータを使用してさまざまなテーマに関する質問に答えることができます。ここでは、Aurora PostgreSQL機械学習機能を利用してAmazon ComprehendやAmazon Bedrockと連携しています。
参考: Build generative AI applications with Amazon Aurora and Knowledge Bases for Amazon Bedrock
Bedrock
aws_bedrock.invoke_model
この関数は、JSON でフォーマットされたテキストを入力として受け取り、Amazon Bedrock でホストされているさまざまなモデルに対して処理し、モデルから JSON テキストレスポンスを返します。このレスポンスには、テキスト、画像、埋め込みが含まれる場合があります。

postgres=> SELECT aws_bedrock.invoke_model(
'amazon.titan-text-express-v1',
'application/json',
'application/json',
'{"inputText": "日本で一番人口の多い都道府県はどこですか?"}'
);
-[ RECORD 1 ]+-------------------------------------------------------------------------------------------------------------------------------------------------
invoke_model | {"inputTextTokenCount":43,"results":[{"tokenCount":40,"outputText":"\n日本で一番人口の多い都道府県は東京都です。","completionReason":"FINISH"}]}
postgres=> SELECT aws_bedrock.invoke_model(
'amazon.titan-text-express-v1',
'application/json',
'application/json',
'{"inputText": "東京の観光地でお勧めは?"}'
);
-[ RECORD 1 ]+----------------------------------------------------------------------------------------------------------------------------------------------------------------------------------
invoke_model | {"inputTextTokenCount":26,"results":[{"tokenCount":74,"outputText":"\n1.浅草寺\n2.東京スカイツリー\n3.上野動物園\n4.靖国神社\n5.国立科学博物館\n…","completionReason":"FINISH"}]}
postgres=>
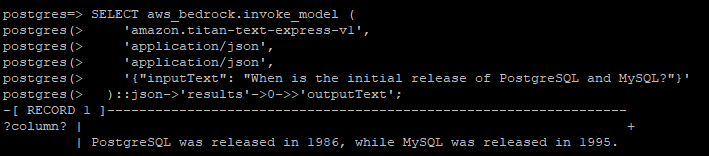
postgres=> SELECT aws_bedrock.invoke_model (
postgres(> 'amazon.titan-text-express-v1',
postgres(> 'application/json',
postgres(> 'application/json',
postgres(> '{"inputText": "When is the initial release of PostgreSQL and MySQL?"}'
postgres(> )::json->'results'->0->>'outputText';
-[ RECORD 1 ]-----------------------------------------------------------------
?column? | +
| PostgreSQL was released in 1986, while MySQL was released in 1995.
aws_bedrock.invoke_model_get_embeddings
モデル出力は、場合によってはベクトル埋め込みを指すことがあります。レスポンスはモデルによって異なるため、別の関数 invoke_model_get_embeddings を使用できます。これは invoke_model とまったく同じように機能しますが、適切な json-key を指定して埋め込みを出力します。
postgres=> SELECT aws_bedrock.invoke_model_get_embeddings(
model_id := 'amazon.titan-embed-text-v1',
content_type := 'application/json',
json_key := 'embedding',
model_input := '{ "inputText": "PostgreSQL I/O monitoring views"}') AS embedding;
-[ RECORD 1 ]-----------------------------------------------------------------------------------------------------------------------------------------------------------------------------
embedding | {-1.0625,-1.1484375,0.578125,-0.08251953,0.39453125,-0.80859375,0.051513672,-0.0016479492,-1.203125,0.49609375,-0.33984375,-0.5546875,-0.1796875,0.07373047,0.33789062,0.47070312,0.296875,-0.5234375,-0.0061950684,0.16210938,-0.6171875,-0.38476562,-0.13867188,0.56640625,-0.2734375,1.265625,0.25585938,0.45117188,-0.64453125,-1.03125,0.4375,1.265625,-0.103027344,0.02758789,<SNIP>
,-0.51953125,-0.94140625,0.40625,0.20898438,-0.20410156,0.984375,-0.37109375,0.053710938,0.17089844,0.16113281,0.44921875,-0.625,-0.15429688,0.32617188,-0.14160156,-0.119628906,-0.061767578,0.671875,0.59375,0.09277344,-0.421875,0.048095703,0.27539062,-0.42773438,-0.9296875,-0.3515625,-0.36914062,1.5546875,1.2421875,0.35546875,-0.5234375,0.3515625,-0.9921875,0.76171875,-0.22167969,-0.16894531,-0.106933594,-0.5703125,-0.26171875,0.625,0.16113281,-0.37695312,-0.16699219,-0.796875,1.21875,-0.36523438}
postgres=>
DB運用中にふとコマンドを忘れてしまった場合にも使えるかもしれません
postgres=> SELECT aws_bedrock.invoke_model (
postgres(> 'amazon.titan-text-express-v1',
postgres(> 'application/json',
postgres(> 'application/json',
postgres(> '{"inputText": "How to confirm current process in PostgreSQL?"}'
postgres(> )::json->'results'->0->>'outputText';
-[ RECORD 1 ]--------------------------------------------------------------------------------------------------
?column? | +
| In PostgreSQL, the "pg_stat_activity" view shows ongoing queries. +
| ```SQL +
| SELECT * +
| FROM pg_stat_activity +
| WHERE pid = my_current_pid(); +
| ``` +
| The "pg_stat_activity" view shows the current state of all active sessions in the PostgreSQL server.
postgres=> select * from pg_stat_activity limit 1;
-[ RECORD 1 ]----+------------------------------
datid |
datname |
pid | 568
leader_pid |
usesysid | 10
usename | rdsadmin
application_name |
client_addr |
client_hostname |
client_port |
backend_start | 2024-03-01 07:01:38.664874+00
xact_start |
query_start |
state_change |
wait_event_type | Activity
wait_event | LogicalLauncherMain
state |
backend_xid |
backend_xmin |
query_id |
query |
backend_type | logical replication launcher
postgres=>
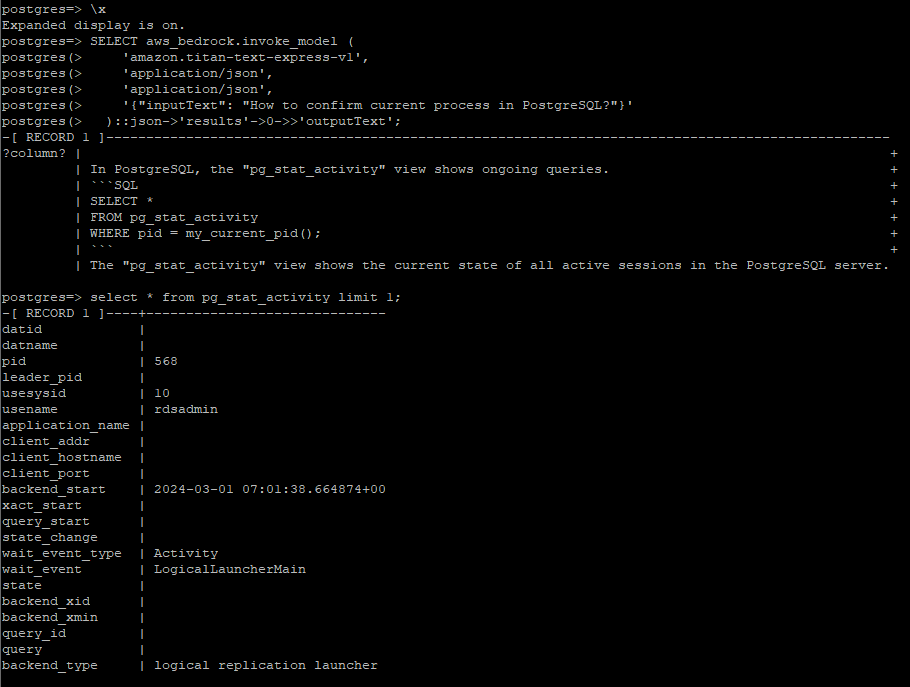
Comprehend
ws_comprehend.detect_sentiment
この関数は、テキストを入力として受け取り、そのテキストの感情的な姿勢がポジティブ、ネガティブ、中立、または混合のいずれであるかを評価します。
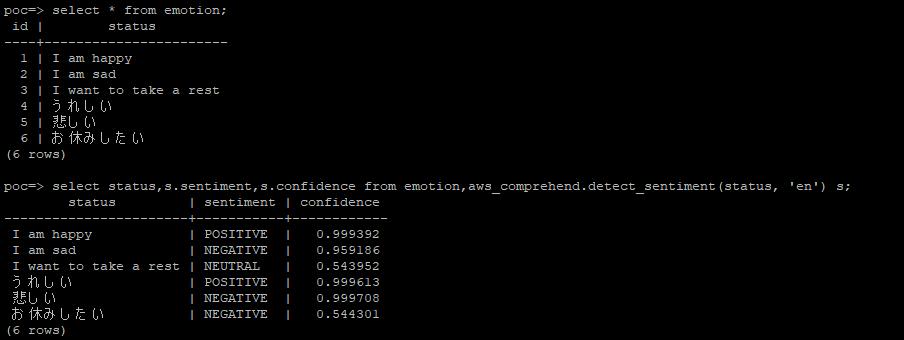
poc=> select status,s.sentiment,s.confidence from emotion,aws_comprehend.detect_sentiment(status, 'en') s;
status | sentiment | confidence
-----------------------+-----------+------------
I am happy | POSITIVE | 0.999392
I am sad | NEGATIVE | 0.959186
I want to take a rest | NEUTRAL | 0.543952
うれしい | POSITIVE | 0.999613
悲しい | NEGATIVE | 0.999708
お休みしたい | NEGATIVE | 0.544301
(6 rows)
参考: Aurora PostgreSQL で Amazon Aurora 機械学習を使用する
上手く使いこなせば、色々なサービスに活用出来そうです。